In
statistics, linear regression is a
linear
Linearity is the property of a mathematical relationship ('' function'') that can be graphically represented as a straight line. Linearity is closely related to '' proportionality''. Examples in physics include rectilinear motion, the linear ...
approach for modelling the relationship between a
scalar response and one or more explanatory variables (also known as
dependent and independent variables). The case of one explanatory variable is called ''
simple linear regression
In statistics, simple linear regression is a linear regression model with a single explanatory variable. That is, it concerns two-dimensional sample points with one independent variable and one dependent variable (conventionally, the ''x'' an ...
''; for more than one, the process is called multiple linear regression.
This term is distinct from
multivariate linear regression
The general linear model or general multivariate regression model is a compact way of simultaneously writing several multiple linear regression models. In that sense it is not a separate statistical linear model. The various multiple linear reg ...
, where multiple
correlated
In statistics, correlation or dependence is any statistical relationship, whether causal or not, between two random variables or bivariate data. Although in the broadest sense, "correlation" may indicate any type of association, in statisti ...
dependent variables are predicted, rather than a single scalar variable.
In linear regression, the relationships are modeled using
linear predictor functions whose unknown model
parameters are
estimated from the
data
In the pursuit of knowledge, data (; ) is a collection of discrete values that convey information, describing quantity, quality, fact, statistics, other basic units of meaning, or simply sequences of symbols that may be further interpret ...
. Such models are called
linear model
In statistics, the term linear model is used in different ways according to the context. The most common occurrence is in connection with regression models and the term is often taken as synonymous with linear regression model. However, the term ...
s. Most commonly, the
conditional mean
In probability theory, the conditional expectation, conditional expected value, or conditional mean of a random variable is its expected value – the value it would take “on average” over an arbitrarily large number of occurrences – given ...
of the response given the values of the explanatory variables (or predictors) is assumed to be an
affine function
In Euclidean geometry, an affine transformation or affinity (from the Latin, ''affinis'', "connected with") is a geometric transformation that preserves lines and parallelism, but not necessarily Euclidean distances and angles.
More genera ...
of those values; less commonly, the conditional
median or some other
quantile is used. Like all forms of
regression analysis
In statistical modeling, regression analysis is a set of statistical processes for estimating the relationships between a dependent variable (often called the 'outcome' or 'response' variable, or a 'label' in machine learning parlance) and one ...
, linear regression focuses on the
conditional probability distribution
In probability theory and statistics, given two jointly distributed random variables X and Y, the conditional probability distribution of Y given X is the probability distribution of Y when X is known to be a particular value; in some cases the c ...
of the response given the values of the predictors, rather than on the
joint probability distribution
Given two random variables that are defined on the same probability space, the joint probability distribution is the corresponding probability distribution on all possible pairs of outputs. The joint distribution can just as well be considere ...
of all of these variables, which is the domain of
multivariate analysis
Multivariate statistics is a subdivision of statistics encompassing the simultaneous observation and analysis of more than one outcome variable.
Multivariate statistics concerns understanding the different aims and background of each of the dif ...
.
Linear regression was the first type of regression analysis to be studied rigorously, and to be used extensively in practical applications. This is because models which depend linearly on their unknown parameters are easier to fit than models which are non-linearly related to their parameters and because the statistical properties of the resulting estimators are easier to determine.
Linear regression has many practical uses. Most applications fall into one of the following two broad categories:
* If the goal is error reduction in
prediction
A prediction (Latin ''præ-'', "before," and ''dicere'', "to say"), or forecast, is a statement about a future event or data. They are often, but not always, based upon experience or knowledge. There is no universal agreement about the exac ...
or
forecasting
Forecasting is the process of making predictions based on past and present data. Later these can be compared (resolved) against what happens. For example, a company might estimate their revenue in the next year, then compare it against the actual ...
, linear regression can be used to fit a predictive model to an observed
data set of values of the response and explanatory variables. After developing such a model, if additional values of the explanatory variables are collected without an accompanying response value, the fitted model can be used to make a prediction of the response.
* If the goal is to explain variation in the response variable that can be attributed to variation in the explanatory variables, linear regression analysis can be applied to quantify the strength of the relationship between the response and the explanatory variables, and in particular to determine whether some explanatory variables may have no linear relationship with the response at all, or to identify which subsets of explanatory variables may contain redundant information about the response.
Linear regression models are often fitted using the
least squares
The method of least squares is a standard approach in regression analysis to approximate the solution of overdetermined systems (sets of equations in which there are more equations than unknowns) by minimizing the sum of the squares of the r ...
approach, but they may also be fitted in other ways, such as by minimizing the "lack of fit" in some other
norm (as with
least absolute deviations
Least absolute deviations (LAD), also known as least absolute errors (LAE), least absolute residuals (LAR), or least absolute values (LAV), is a statistical optimality criterion and a statistical optimization technique based minimizing the '' su ...
regression), or by minimizing a penalized version of the least squares
cost function as in
ridge regression
Ridge regression is a method of estimating the coefficients of multiple- regression models in scenarios where the independent variables are highly correlated. It has been used in many fields including econometrics, chemistry, and engineering. Also ...
(''L''
2-norm penalty) and
lasso
A lasso ( or ), also called lariat, riata, or reata (all from Castilian, la reata 're-tied rope'), is a loop of rope designed as a restraint to be thrown around a target and tightened when pulled. It is a well-known tool of the Spanish a ...
(''L''
1-norm penalty). Conversely, the least squares approach can be used to fit models that are not linear models. Thus, although the terms "least squares" and "linear model" are closely linked, they are not synonymous.
Formulation

Given a
data
In the pursuit of knowledge, data (; ) is a collection of discrete values that convey information, describing quantity, quality, fact, statistics, other basic units of meaning, or simply sequences of symbols that may be further interpret ...
set
of ''n''
statistical units, a linear regression model assumes that the relationship between the dependent variable ''y'' and the
''p''-vector of regressors x is
linear
Linearity is the property of a mathematical relationship ('' function'') that can be graphically represented as a straight line. Linearity is closely related to '' proportionality''. Examples in physics include rectilinear motion, the linear ...
. This relationship is modeled through a ''disturbance term'' or ''error variable'' ''ε'' — an unobserved
random variable
A random variable (also called random quantity, aleatory variable, or stochastic variable) is a mathematical formalization of a quantity or object which depends on random events. It is a mapping or a function from possible outcomes (e.g., the p ...
that adds "noise" to the linear relationship between the dependent variable and regressors. Thus the model takes the form
:
where
T denotes the
transpose
In linear algebra, the transpose of a matrix is an operator which flips a matrix over its diagonal;
that is, it switches the row and column indices of the matrix by producing another matrix, often denoted by (among other notations).
The tr ...
, so that x
''i''T''β'' is the
inner product
In mathematics, an inner product space (or, rarely, a Hausdorff pre-Hilbert space) is a real vector space or a complex vector space with an operation called an inner product. The inner product of two vectors in the space is a scalar, often ...
between
vectors x
''i'' and ''β''.
Often these ''n'' equations are stacked together and written in
matrix notation as
:
where
:
:
:
Notation and terminology
*
is a vector of observed values
of the variable called the ''regressand'', ''endogenous variable'', ''response variable'', ''measured variable'', ''criterion variable'', or ''
dependent variable
Dependent and independent variables are variables in mathematical modeling, statistical modeling and experimental sciences. Dependent variables receive this name because, in an experiment, their values are studied under the supposition or dema ...
''. This variable is also sometimes known as the ''predicted variable'', but this should not be confused with ''predicted values'', which are denoted
. The decision as to which variable in a data set is modeled as the dependent variable and which are modeled as the independent variables may be based on a presumption that the value of one of the variables is caused by, or directly influenced by the other variables. Alternatively, there may be an operational reason to model one of the variables in terms of the others, in which case there need be no presumption of causality.
*
may be seen as a matrix of row-vectors
or of ''n''-dimensional column-vectors
, which are known as ''regressors'', ''exogenous variables'', ''explanatory variables'', ''covariates'', ''input variables'', ''predictor variables'', or ''
independent variables'' (not to be confused with the concept of
independent random variables). The matrix
is sometimes called the
design matrix
In statistics and in particular in regression analysis, a design matrix, also known as model matrix or regressor matrix and often denoted by X, is a matrix of values of explanatory variables of a set of objects. Each row represents an individual ...
.
** Usually a constant is included as one of the regressors. In particular,
for
. The corresponding element of ''β'' is called the ''
intercept''. Many statistical inference procedures for linear models require an intercept to be present, so it is often included even if theoretical considerations suggest that its value should be zero.
** Sometimes one of the regressors can be a non-linear function of another regressor or of the data, as in
polynomial regression
In statistics, polynomial regression is a form of regression analysis in which the relationship between the independent variable ''x'' and the dependent variable ''y'' is modelled as an ''n''th degree polynomial in ''x''. Polynomial regression fi ...
and
segmented regression. The model remains linear as long as it is linear in the parameter vector ''β''.
** The values ''x''
''ij'' may be viewed as either observed values of
random variable
A random variable (also called random quantity, aleatory variable, or stochastic variable) is a mathematical formalization of a quantity or object which depends on random events. It is a mapping or a function from possible outcomes (e.g., the p ...
s ''X''
''j'' or as fixed values chosen prior to observing the dependent variable. Both interpretations may be appropriate in different cases, and they generally lead to the same estimation procedures; however different approaches to asymptotic analysis are used in these two situations.
*
is a
-dimensional ''parameter vector'', where
is the intercept term (if one is included in the model—otherwise
is ''p''-dimensional). Its elements are known as ''effects'' or ''regression coefficients'' (although the latter term is sometimes reserved for the ''estimated'' effects). In
simple linear regression
In statistics, simple linear regression is a linear regression model with a single explanatory variable. That is, it concerns two-dimensional sample points with one independent variable and one dependent variable (conventionally, the ''x'' an ...
, ''p''=1, and the coefficient is known as ''regression slope''. Statistical
estimation
Estimation (or estimating) is the process of finding an estimate or approximation, which is a value that is usable for some purpose even if input data may be incomplete, uncertain, or unstable. The value is nonetheless usable because it is de ...
and
inference
Inferences are steps in reasoning, moving from premises to logical consequences; etymologically, the word '' infer'' means to "carry forward". Inference is theoretically traditionally divided into deduction and induction, a distinction that ...
in linear regression focuses on ''β''. The elements of this parameter vector are interpreted as the
partial derivative
In mathematics, a partial derivative of a function of several variables is its derivative with respect to one of those variables, with the others held constant (as opposed to the total derivative, in which all variables are allowed to vary). Pa ...
s of the dependent variable with respect to the various independent variables.
*
is a vector of values
. This part of the model is called the ''error term'', ''disturbance term'', or sometimes ''noise'' (in contrast with the "signal" provided by the rest of the model). This variable captures all other factors which influence the dependent variable ''y'' other than the regressors x. The relationship between the error term and the regressors, for example their
correlation
In statistics, correlation or dependence is any statistical relationship, whether causal or not, between two random variables or bivariate data. Although in the broadest sense, "correlation" may indicate any type of association, in statisti ...
, is a crucial consideration in formulating a linear regression model, as it will determine the appropriate estimation method.
Fitting a linear model to a given data set usually requires estimating the regression coefficients
such that the error term
is minimized. For example, it is common to use the sum of squared errors
as a measure of
for minimization.
Example
Consider a situation where a small ball is being tossed up in the air and then we measure its heights of ascent ''h
i'' at various moments in time ''t
i''. Physics tells us that, ignoring the drag, the relationship can be modeled as
:
where ''β''
1 determines the initial velocity of the ball, ''β''
2 is proportional to the
standard gravity
The standard acceleration due to gravity (or standard acceleration of free fall), sometimes abbreviated as standard gravity, usually denoted by or , is the nominal gravitational acceleration of an object in a vacuum near the surface of the Earth ...
, and ''ε''
''i'' is due to measurement errors. Linear regression can be used to estimate the values of ''β''
1 and ''β''
2 from the measured data. This model is non-linear in the time variable, but it is linear in the parameters ''β''
1 and ''β''
2; if we take regressors x
''i'' = (''x''
''i''1, ''x''
''i''2) = (''t''
''i'', ''t''
''i''2), the model takes on the standard form
:
Assumptions
Standard linear regression models with standard estimation techniques make a number of assumptions about the predictor variables, the response variables and their relationship. Numerous extensions have been developed that allow each of these assumptions to be relaxed (i.e. reduced to a weaker form), and in some cases eliminated entirely. Generally these extensions make the estimation procedure more complex and time-consuming, and may also require more data in order to produce an equally precise model.
The following are the major assumptions made by standard linear regression models with standard estimation techniques (e.g.
ordinary least squares
In statistics, ordinary least squares (OLS) is a type of linear least squares method for choosing the unknown parameters in a linear regression model (with fixed level-one effects of a linear function of a set of explanatory variables) by the ...
):
*Weak exogeneity. This essentially means that the predictor variables ''x'' can be treated as fixed values, rather than
random variable
A random variable (also called random quantity, aleatory variable, or stochastic variable) is a mathematical formalization of a quantity or object which depends on random events. It is a mapping or a function from possible outcomes (e.g., the p ...
s. This means, for example, that the predictor variables are assumed to be error-free—that is, not contaminated with measurement errors. Although this assumption is not realistic in many settings, dropping it leads to significantly more difficult
errors-in-variables models.
*Linearity. This means that the mean of the response variable is a
linear combination of the parameters (regression coefficients) and the predictor variables. Note that this assumption is much less restrictive than it may at first seem. Because the predictor variables are treated as fixed values (see above), linearity is really only a restriction on the parameters. The predictor variables themselves can be arbitrarily transformed, and in fact multiple copies of the same underlying predictor variable can be added, each one transformed differently. This technique is used, for example, in
polynomial regression
In statistics, polynomial regression is a form of regression analysis in which the relationship between the independent variable ''x'' and the dependent variable ''y'' is modelled as an ''n''th degree polynomial in ''x''. Polynomial regression fi ...
, which uses linear regression to fit the response variable as an arbitrary
polynomial
In mathematics, a polynomial is an expression consisting of indeterminates (also called variables) and coefficients, that involves only the operations of addition, subtraction, multiplication, and positive-integer powers of variables. An ex ...
function (up to a given degree) of a predictor variable. With this much flexibility, models such as polynomial regression often have "too much power", in that they tend to
overfit the data. As a result, some kind of
regularization must typically be used to prevent unreasonable solutions coming out of the estimation process. Common examples are
ridge regression
Ridge regression is a method of estimating the coefficients of multiple- regression models in scenarios where the independent variables are highly correlated. It has been used in many fields including econometrics, chemistry, and engineering. Also ...
and
lasso regression.
Bayesian linear regression
Bayesian linear regression is a type of conditional modeling in which the mean of one variable is described by a linear combination of other variables, with the goal of obtaining the posterior probability of the regression coefficients (as wel ...
can also be used, which by its nature is more or less immune to the problem of overfitting. (In fact,
ridge regression
Ridge regression is a method of estimating the coefficients of multiple- regression models in scenarios where the independent variables are highly correlated. It has been used in many fields including econometrics, chemistry, and engineering. Also ...
and
lasso regression can both be viewed as special cases of Bayesian linear regression, with particular types of
prior distributions placed on the regression coefficients.)
*Constant variance (a.k.a.
homoscedasticity
In statistics, a sequence (or a vector) of random variables is homoscedastic () if all its random variables have the same finite variance. This is also known as homogeneity of variance. The complementary notion is called heteroscedasticity. The s ...
). This means that the variance of the errors does not depend on the values of the predictor variables. Thus the variability of the responses for given fixed values of the predictors is the same regardless of how large or small the responses are. This is often not the case, as a variable whose mean is large will typically have a greater variance than one whose mean is small. For example, a person whose income is predicted to be $100,000 may easily have an actual income of $80,000 or $120,000—i.e., a
standard deviation of around $20,000—while another person with a predicted income of $10,000 is unlikely to have the same $20,000 standard deviation, since that would imply their actual income could vary anywhere between −$10,000 and $30,000. (In fact, as this shows, in many cases—often the same cases where the assumption of normally distributed errors fails—the variance or standard deviation should be predicted to be proportional to the mean, rather than constant.) The absence of homoscedasticity is called
heteroscedasticity. In order to check this assumption, a plot of residuals versus predicted values (or the values of each individual predictor) can be examined for a "fanning effect" (i.e., increasing or decreasing vertical spread as one moves left to right on the plot). A plot of the absolute or squared residuals versus the predicted values (or each predictor) can also be examined for a trend or curvature. Formal tests can also be used; see
Heteroscedasticity. The presence of heteroscedasticity will result in an overall "average" estimate of variance being used instead of one that takes into account the true variance structure. This leads to less precise (but in the case of
ordinary least squares
In statistics, ordinary least squares (OLS) is a type of linear least squares method for choosing the unknown parameters in a linear regression model (with fixed level-one effects of a linear function of a set of explanatory variables) by the ...
, not biased) parameter estimates and biased standard errors, resulting in misleading tests and interval estimates. The
mean squared error
In statistics, the mean squared error (MSE) or mean squared deviation (MSD) of an estimator (of a procedure for estimating an unobserved quantity) measures the average of the squares of the errors—that is, the average squared difference betwe ...
for the model will also be wrong. Various estimation techniques including
weighted least squares
Weighted least squares (WLS), also known as weighted linear regression, is a generalization of ordinary least squares and linear regression in which knowledge of the variance of observations is incorporated into the regression.
WLS is also a speci ...
and the use of
heteroscedasticity-consistent standard errors can handle heteroscedasticity in a quite general way.
Bayesian linear regression
Bayesian linear regression is a type of conditional modeling in which the mean of one variable is described by a linear combination of other variables, with the goal of obtaining the posterior probability of the regression coefficients (as wel ...
techniques can also be used when the variance is assumed to be a function of the mean. It is also possible in some cases to fix the problem by applying a transformation to the response variable (e.g., fitting the
logarithm
In mathematics, the logarithm is the inverse function to exponentiation. That means the logarithm of a number to the base is the exponent to which must be raised, to produce . For example, since , the ''logarithm base'' 10 of ...
of the response variable using a linear regression model, which implies that the response variable itself has a
log-normal distribution
In probability theory, a log-normal (or lognormal) distribution is a continuous probability distribution of a random variable whose logarithm is normally distributed. Thus, if the random variable is log-normally distributed, then has a normal ...
rather than a
normal distribution
In statistics, a normal distribution or Gaussian distribution is a type of continuous probability distribution for a real-valued random variable. The general form of its probability density function is
:
f(x) = \frac e^
The parameter \mu i ...
).
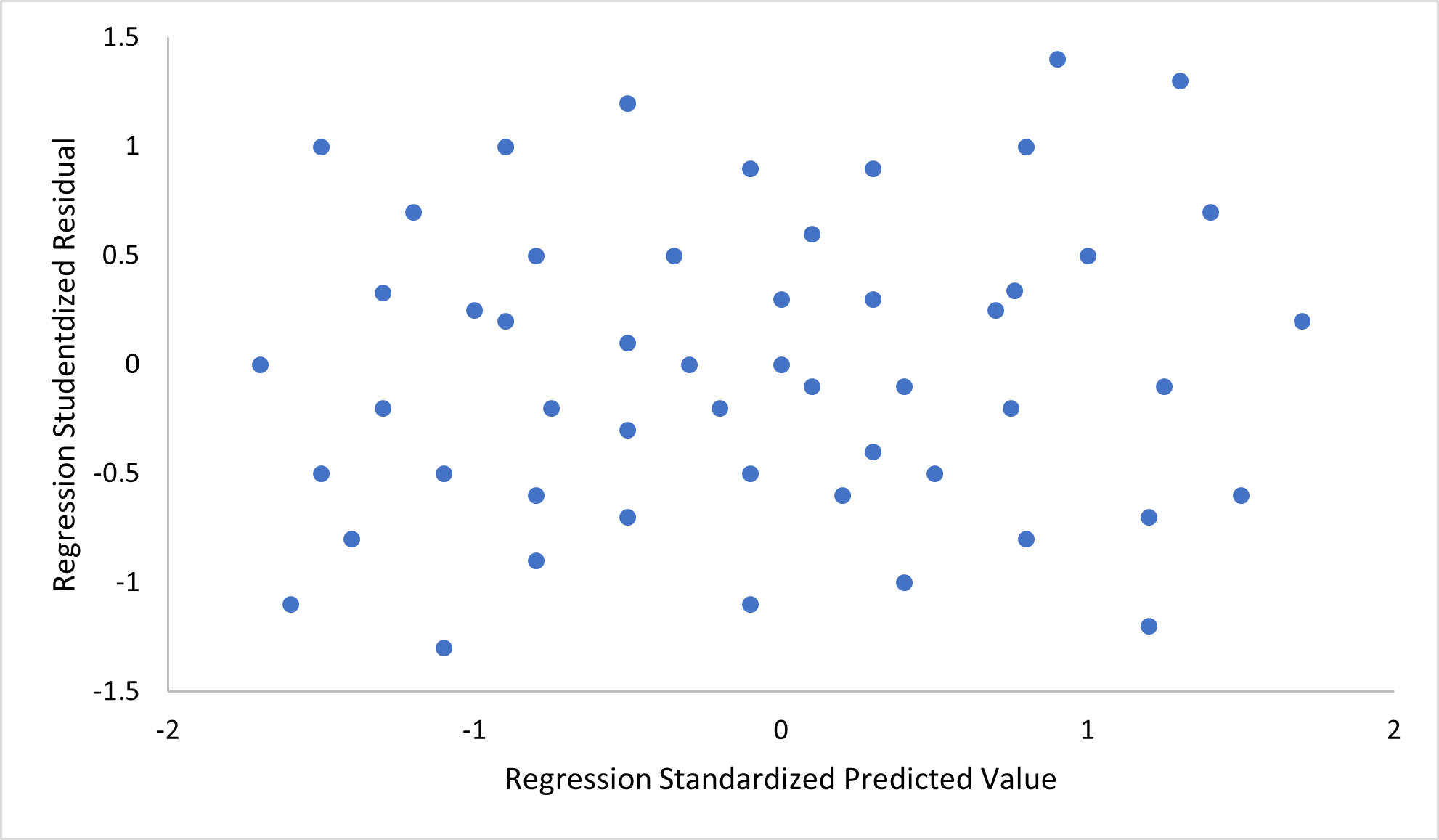
* Independence of errors. This assumes that the errors of the response variables are uncorrelated with each other. (Actual
statistical independence is a stronger condition than mere lack of correlation and is often not needed, although it can be exploited if it is known to hold.) Some methods such as
generalized least squares are capable of handling correlated errors, although they typically require significantly more data unless some sort of
regularization is used to bias the model towards assuming uncorrelated errors.
Bayesian linear regression
Bayesian linear regression is a type of conditional modeling in which the mean of one variable is described by a linear combination of other variables, with the goal of obtaining the posterior probability of the regression coefficients (as wel ...
is a general way of handling this issue.
* Lack of perfect multicollinearity in the predictors. For standard
least squares
The method of least squares is a standard approach in regression analysis to approximate the solution of overdetermined systems (sets of equations in which there are more equations than unknowns) by minimizing the sum of the squares of the r ...
estimation methods, the design matrix ''X'' must have full
column rank ''p''; otherwise perfect
multicollinearity exists in the predictor variables, meaning a linear relationship exists between two or more predictor variables. This can be caused by accidentally duplicating a variable in the data, using a linear transformation of a variable along with the original (e.g., the same temperature measurements expressed in Fahrenheit and Celsius), or including a linear combination of multiple variables in the model, such as their mean. It can also happen if there is too little data available compared to the number of parameters to be estimated (e.g., fewer data points than regression coefficients). Near violations of this assumption, where predictors are highly but not perfectly correlated, can reduce the precision of parameter estimates (see
Variance inflation factor). In the case of perfect multicollinearity, the parameter vector ''β'' will be
non-identifiable
In statistics, identifiability is a property which a model must satisfy for precise inference to be possible. A model is identifiable if it is theoretically possible to learn the true values of this model's underlying parameters after obtaining an ...
—it has no unique solution. In such a case, only some of the parameters can be identified (i.e., their values can only be estimated within some linear subspace of the full parameter space R
''p''). See
partial least squares regression. Methods for fitting linear models with multicollinearity have been developed,
some of which require additional assumptions such as "effect sparsity"—that a large fraction of the effects are exactly zero. Note that the more computationally expensive iterated algorithms for parameter estimation, such as those used in
generalized linear model
In statistics, a generalized linear model (GLM) is a flexible generalization of ordinary linear regression. The GLM generalizes linear regression by allowing the linear model to be related to the response variable via a ''link function'' and by ...
s, do not suffer from this problem.
Beyond these assumptions, several other statistical properties of the data strongly influence the performance of different estimation methods:
* The statistical relationship between the error terms and the regressors plays an important role in determining whether an estimation procedure has desirable sampling properties such as being unbiased and consistent.
* The arrangement, or
probability distribution
In probability theory and statistics, a probability distribution is the mathematical function that gives the probabilities of occurrence of different possible outcomes for an experiment. It is a mathematical description of a random phenomeno ...
of the predictor variables x has a major influence on the precision of estimates of ''β''.
Sampling and
design of experiments
The design of experiments (DOE, DOX, or experimental design) is the design of any task that aims to describe and explain the variation of information under conditions that are hypothesized to reflect the variation. The term is generally associ ...
are highly developed subfields of statistics that provide guidance for collecting data in such a way to achieve a precise estimate of ''β''.
Interpretation

A fitted linear regression model can be used to identify the relationship between a single predictor variable ''x''
''j'' and the response variable ''y'' when all the other predictor variables in the model are "held fixed". Specifically, the interpretation of ''β''
''j'' is the
expected change in ''y'' for a one-unit change in ''x''
''j'' when the other covariates are held fixed—that is, the expected value of the
partial derivative
In mathematics, a partial derivative of a function of several variables is its derivative with respect to one of those variables, with the others held constant (as opposed to the total derivative, in which all variables are allowed to vary). Pa ...
of ''y'' with respect to ''x''
''j''. This is sometimes called the ''unique effect'' of ''x''
''j'' on ''y''. In contrast, the ''marginal effect'' of ''x''
''j'' on ''y'' can be assessed using a
correlation coefficient
A correlation coefficient is a numerical measure of some type of correlation, meaning a statistical relationship between two variables. The variables may be two columns of a given data set of observations, often called a sample, or two componen ...
or
simple linear regression
In statistics, simple linear regression is a linear regression model with a single explanatory variable. That is, it concerns two-dimensional sample points with one independent variable and one dependent variable (conventionally, the ''x'' an ...
model relating only ''x''
''j'' to ''y''; this effect is the
total derivative
In mathematics, the total derivative of a function at a point is the best linear approximation near this point of the function with respect to its arguments. Unlike partial derivatives, the total derivative approximates the function with r ...
of ''y'' with respect to ''x''
''j''.
Care must be taken when interpreting regression results, as some of the regressors may not allow for marginal changes (such as
dummy variables, or the intercept term), while others cannot be held fixed (recall the example from the introduction: it would be impossible to "hold ''t
i'' fixed" and at the same time change the value of ''t
i''
2).
It is possible that the unique effect can be nearly zero even when the marginal effect is large. This may imply that some other covariate captures all the information in ''x''
''j'', so that once that variable is in the model, there is no contribution of ''x''
''j'' to the variation in ''y''. Conversely, the unique effect of ''x''
''j'' can be large while its marginal effect is nearly zero. This would happen if the other covariates explained a great deal of the variation of ''y'', but they mainly explain variation in a way that is complementary to what is captured by ''x''
''j''. In this case, including the other variables in the model reduces the part of the variability of ''y'' that is unrelated to ''x''
''j'', thereby strengthening the apparent relationship with ''x''
''j''.
The meaning of the expression "held fixed" may depend on how the values of the predictor variables arise. If the experimenter directly sets the values of the predictor variables according to a study design, the comparisons of interest may literally correspond to comparisons among units whose predictor variables have been "held fixed" by the experimenter. Alternatively, the expression "held fixed" can refer to a selection that takes place in the context of data analysis. In this case, we "hold a variable fixed" by restricting our attention to the subsets of the data that happen to have a common value for the given predictor variable. This is the only interpretation of "held fixed" that can be used in an observational study.
The notion of a "unique effect" is appealing when studying a complex system where multiple interrelated components influence the response variable. In some cases, it can literally be interpreted as the causal effect of an intervention that is linked to the value of a predictor variable. However, it has been argued that in many cases multiple regression analysis fails to clarify the relationships between the predictor variables and the response variable when the predictors are correlated with each other and are not assigned following a study design.
Group effects
In a multiple linear regression model
:
parameter
of predictor variable
represents the individual effect of
. It has an interpretation as the expected change in the response variable
when
increases by one unit with other predictor variables held constant. When
is strongly correlated with other predictor variables, it is improbable that
can increase by one unit with other variables held constant. In this case, the interpretation of
becomes problematic as it is based on an improbable condition, and the effect of
cannot be evaluated in isolation.
For a group of predictor variables, say,
, a group effect
is defined as a linear combination of their parameters
:
where
is a weight vector satisfying
. Because of the constraint on
,
is also referred to as a normalized group effect. A group effect
has an interpretation as the expected change in
when variables in the group
change by the amount
, respectively, at the same time with variables not in the group held constant. It generalizes the individual effect of a variable to a group of variables in that (
) if
, then the group effect reduces to an individual effect, and (
) if
and
for
, then the group effect also reduces to an individual effect.
A group effect
is said to be meaningful if the underlying simultaneous changes of the
variables
is probable.
Group effects provide a means to study the collective impact of strongly correlated predictor variables in linear regression models. Individual effects of such variables are not well-defined as their parameters do not have good interpretations. Furthermore, when the sample size is not large, none of their parameters can be accurately estimated by the
least squares regression due to the
multicollinearity problem. Nevertheless, there are meaningful group effects that have good interpretations and can be accurately estimated by the least squares regression. A simple way to identify these meaningful group effects is to use an all positive correlations (APC) arrangement of the strongly correlated variables under which pairwise correlations among these variables are all positive, and standardize all
predictor variables in the model so that they all have mean zero and length one. To illustrate this, suppose that
is a group of strongly correlated variables in an APC arrangement and that they are not strongly correlated with predictor variables outside the group. Let
be the centred
and
be the standardized
. Then, the standardized linear regression model is
:
Parameters
in the original model, including
, are simple functions of
in the standardized model. The standardization of variables does not change their correlations, so
is a group of strongly correlated variables in an APC arrangement and they are not strongly correlated with other predictor variables in the standardized model. A group effect of
is
:
and its minimum-variance unbiased linear estimator is
:
where
is the least squares estimator of
. In particular, the average group effect of the
standardized variables is
:
which has an interpretation as the expected change in
when all
in the strongly correlated group increase by
th of a unit at the same time with variables outside the group held constant. With strong positive correlations and in standardized units, variables in the group are approximately equal, so they are likely to increase at the same time and in similar amount. Thus, the average group effect
is a meaningful effect. It can be accurately estimated by its minimum-variance unbiased linear estimator
, even when individually none of the
can be accurately estimated by
.
Not all group effects are meaningful or can be accurately estimated. For example,
is a special group effect with weights
and
for
, but it cannot be accurately estimated by
. It is also not a meaningful effect. In general, for a group of
strongly correlated predictor variables in an APC arrangement in the standardized model, group effects whose weight vectors
are at or near the centre of the simplex
(
) are meaningful and can be accurately estimated by their minimum-variance unbiased linear estimators. Effects with weight vectors far away from the centre are not meaningful as such weight vectors represent simultaneous changes of the variables that violate the strong positive correlations of the standardized variables in an APC arrangement. As such, they are not probable. These effects also cannot be accurately estimated.
Applications of the group effects include (1) estimation and inference for meaningful group effects on the response variable, (2) testing for "group significance" of the
variables via testing
versus
, and (3) characterizing the region of the predictor variable space over which predictions by the least squares estimated model are accurate.
A group effect of the original variables
can be expressed as a constant times a group effect of the standardized variables
. The former is meaningful when the latter is. Thus meaningful group effects of the original variables can be found through meaningful group effects of the standardized variables.
Extensions
Numerous extensions of linear regression have been developed, which allow some or all of the assumptions underlying the basic model to be relaxed.
Simple and multiple linear regression
The very simplest case of a single
scalar predictor variable ''x'' and a single scalar response variable ''y'' is known as ''
simple linear regression
In statistics, simple linear regression is a linear regression model with a single explanatory variable. That is, it concerns two-dimensional sample points with one independent variable and one dependent variable (conventionally, the ''x'' an ...
''. The extension to multiple and/or
vector-valued predictor variables (denoted with a capital ''X'') is known as multiple linear regression, also known as multivariable linear regression (not to be confused with ''
multivariate linear regression
The general linear model or general multivariate regression model is a compact way of simultaneously writing several multiple linear regression models. In that sense it is not a separate statistical linear model. The various multiple linear reg ...
'').
Multiple linear regression is a generalization of
simple linear regression
In statistics, simple linear regression is a linear regression model with a single explanatory variable. That is, it concerns two-dimensional sample points with one independent variable and one dependent variable (conventionally, the ''x'' an ...
to the case of more than one independent variable, and a
special case of general linear models, restricted to one dependent variable. The basic model for multiple linear regression is
:
for each observation ''i'' = 1, ... , ''n''.
In the formula above we consider ''n'' observations of one dependent variable and ''p'' independent variables. Thus, ''Y''
''i'' is the ''i''
th observation of the dependent variable, ''X''
''ij'' is ''i''
th observation of the ''j''
th independent variable, ''j'' = 1, 2, ..., ''p''. The values ''β''
''j'' represent parameters to be estimated, and ''ε''
''i'' is the ''i''
th independent identically distributed normal error.
In the more general multivariate linear regression, there is one equation of the above form for each of ''m'' > 1 dependent variables that share the same set of explanatory variables and hence are estimated simultaneously with each other:
:
for all observations indexed as ''i'' = 1, ... , ''n'' and for all dependent variables indexed as ''j = 1, ... , ''m''.
Nearly all real-world regression models involve multiple predictors, and basic descriptions of linear regression are often phrased in terms of the multiple regression model. Note, however, that in these cases the response variable ''y'' is still a scalar. Another term, ''multivariate linear regression'', refers to cases where ''y'' is a vector, i.e., the same as ''general linear regression''.
General linear models
The
general linear model
The general linear model or general multivariate regression model is a compact way of simultaneously writing several multiple linear regression models. In that sense it is not a separate statistical linear model. The various multiple linear regr ...
considers the situation when the response variable is not a scalar (for each observation) but a vector, y
''i''. Conditional linearity of
is still assumed, with a matrix ''B'' replacing the vector ''β'' of the classical linear regression model. Multivariate analogues of
ordinary least squares
In statistics, ordinary least squares (OLS) is a type of linear least squares method for choosing the unknown parameters in a linear regression model (with fixed level-one effects of a linear function of a set of explanatory variables) by the ...
(OLS) and
generalized least squares (GLS) have been developed. "General linear models" are also called "multivariate linear models". These are not the same as multivariable linear models (also called "multiple linear models").
Heteroscedastic models
Various models have been created that allow for
heteroscedasticity, i.e. the errors for different response variables may have different
variance
In probability theory and statistics, variance is the expectation of the squared deviation of a random variable from its population mean or sample mean. Variance is a measure of dispersion, meaning it is a measure of how far a set of number ...
s. For example,
weighted least squares
Weighted least squares (WLS), also known as weighted linear regression, is a generalization of ordinary least squares and linear regression in which knowledge of the variance of observations is incorporated into the regression.
WLS is also a speci ...
is a method for estimating linear regression models when the response variables may have different error variances, possibly with correlated errors. (See also
Weighted linear least squares, and
Generalized least squares.)
Heteroscedasticity-consistent standard errors is an improved method for use with uncorrelated but potentially heteroscedastic errors.
Generalized linear models
Generalized linear model
In statistics, a generalized linear model (GLM) is a flexible generalization of ordinary linear regression. The GLM generalizes linear regression by allowing the linear model to be related to the response variable via a ''link function'' and by ...
s (GLMs) are a framework for modeling response variables that are bounded or discrete. This is used, for example:
*when modeling positive quantities (e.g. prices or populations) that vary over a large scale—which are better described using a
skewed distribution such as the
log-normal distribution
In probability theory, a log-normal (or lognormal) distribution is a continuous probability distribution of a random variable whose logarithm is normally distributed. Thus, if the random variable is log-normally distributed, then has a normal ...
or
Poisson distribution
In probability theory and statistics, the Poisson distribution is a discrete probability distribution that expresses the probability of a given number of events occurring in a fixed interval of time or space if these events occur with a known ...
(although GLMs are not used for log-normal data, instead the response variable is simply transformed using the logarithm function);
*when modeling
categorical data, such as the choice of a given candidate in an election (which is better described using a
Bernoulli distribution
In probability theory and statistics, the Bernoulli distribution, named after Swiss mathematician Jacob Bernoulli,James Victor Uspensky: ''Introduction to Mathematical Probability'', McGraw-Hill, New York 1937, page 45 is the discrete probab ...
/
binomial distribution
In probability theory and statistics, the binomial distribution with parameters ''n'' and ''p'' is the discrete probability distribution of the number of successes in a sequence of ''n'' independent experiments, each asking a yes–no qu ...
for binary choices, or a
categorical distribution/
multinomial distribution
In probability theory, the multinomial distribution is a generalization of the binomial distribution. For example, it models the probability of counts for each side of a ''k''-sided dice rolled ''n'' times. For ''n'' independent trials each of w ...
for multi-way choices), where there are a fixed number of choices that cannot be meaningfully ordered;
*when modeling
ordinal data, e.g. ratings on a scale from 0 to 5, where the different outcomes can be ordered but where the quantity itself may not have any absolute meaning (e.g. a rating of 4 may not be "twice as good" in any objective sense as a rating of 2, but simply indicates that it is better than 2 or 3 but not as good as 5).
Generalized linear models allow for an arbitrary ''link function'', ''g'', that relates the
mean
There are several kinds of mean in mathematics, especially in statistics. Each mean serves to summarize a given group of data, often to better understand the overall value ( magnitude and sign) of a given data set.
For a data set, the '' ari ...
of the response variable(s) to the predictors:
. The link function is often related to the distribution of the response, and in particular it typically has the effect of transforming between the
range of the linear predictor and the range of the response variable.
Some common examples of GLMs are:
*
Poisson regression
In statistics, Poisson regression is a generalized linear model form of regression analysis used to model count data and contingency tables. Poisson regression assumes the response variable ''Y'' has a Poisson distribution, and assumes the loga ...
for count data.
*
Logistic regression
In statistics, the logistic model (or logit model) is a statistical model that models the probability of an event taking place by having the log-odds for the event be a linear function (calculus), linear combination of one or more independent var ...
and
probit regression
In statistics, a probit model is a type of regression where the dependent variable can take only two values, for example married or not married. The word is a portmanteau, coming from ''probability'' + ''unit''. The purpose of the model is to est ...
for binary data.
*
Multinomial logistic regression
In statistics, multinomial logistic regression is a statistical classification, classification method that generalizes logistic regression to multiclass classification, multiclass problems, i.e. with more than two possible discrete outcomes. T ...
and
multinomial probit regression for categorical data.
*
Ordered logit
In statistics, the ordered logit model (also ordered logistic regression or proportional odds model) is an ordinal regression model—that is, a regression model for ordinal dependent variables—first considered by Peter McCullagh. For exampl ...
and
ordered probit regression for ordinal data.
Single index models allow some degree of nonlinearity in the relationship between ''x'' and ''y'', while preserving the central role of the linear predictor ''β''′''x'' as in the classical linear regression model. Under certain conditions, simply applying OLS to data from a single-index model will consistently estimate ''β'' up to a proportionality constant.
Hierarchical linear models
Hierarchical linear models (or ''multilevel regression'') organizes the data into a hierarchy of regressions, for example where ''A'' is regressed on ''B'', and ''B'' is regressed on ''C''. It is often used where the variables of interest have a natural hierarchical structure such as in educational statistics, where students are nested in classrooms, classrooms are nested in schools, and schools are nested in some administrative grouping, such as a school district. The response variable might be a measure of student achievement such as a test score, and different covariates would be collected at the classroom, school, and school district levels.
Errors-in-variables
Errors-in-variables models (or "measurement error models") extend the traditional linear regression model to allow the predictor variables ''X'' to be observed with error. This error causes standard estimators of ''β'' to become biased. Generally, the form of bias is an attenuation, meaning that the effects are biased toward zero.
Others
* In
Dempster–Shafer theory
The theory of belief functions, also referred to as evidence theory or Dempster–Shafer theory (DST), is a general framework for reasoning with uncertainty, with understood connections to other frameworks such as probability, possibility and i ...
, or a
linear belief function in particular, a linear regression model may be represented as a partially swept matrix, which can be combined with similar matrices representing observations and other assumed normal distributions and state equations. The combination of swept or unswept matrices provides an alternative method for estimating linear regression models.
Estimation methods
A large number of procedures have been developed for
parameter
A parameter (), generally, is any characteristic that can help in defining or classifying a particular system (meaning an event, project, object, situation, etc.). That is, a parameter is an element of a system that is useful, or critical, when ...
estimation and inference in linear regression. These methods differ in computational simplicity of algorithms, presence of a closed-form solution, robustness with respect to heavy-tailed distributions, and theoretical assumptions needed to validate desirable statistical properties such as
consistency
In classical deductive logic, a consistent theory is one that does not lead to a logical contradiction. The lack of contradiction can be defined in either semantic or syntactic terms. The semantic definition states that a theory is consistent ...
and asymptotic
efficiency.
Some of the more common estimation techniques for linear regression are summarized below.
Least-squares estimation and related techniques
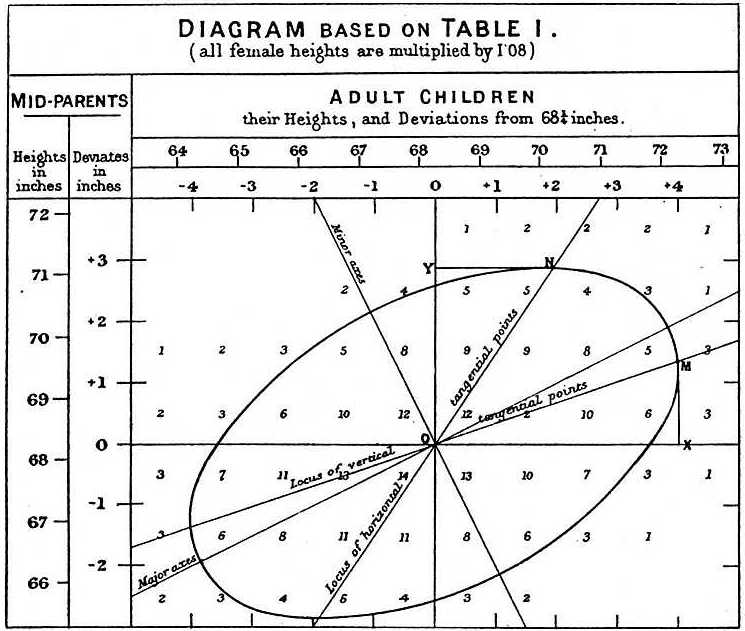
Assuming that the independent variable is